Ari Zelmanow on how researchers can lead in an era of AI & automation
AI is reshaping the research landscape – but it’s not replacing researchers. Instead, it’s giving them superpowers. So, how can Research and Research Ops professionals harness AI to maximize their impact? And what unique value do researchers bring that AI can’t replicate?
Ari Zelmanow, Research Leader at Twilio, joined us for a Rally AMA on February 20 for an exciting discussion to answer these questions.
Missed the event? Or want to revisit the key takeaways? Read the full recap below or watch the live discussion here.
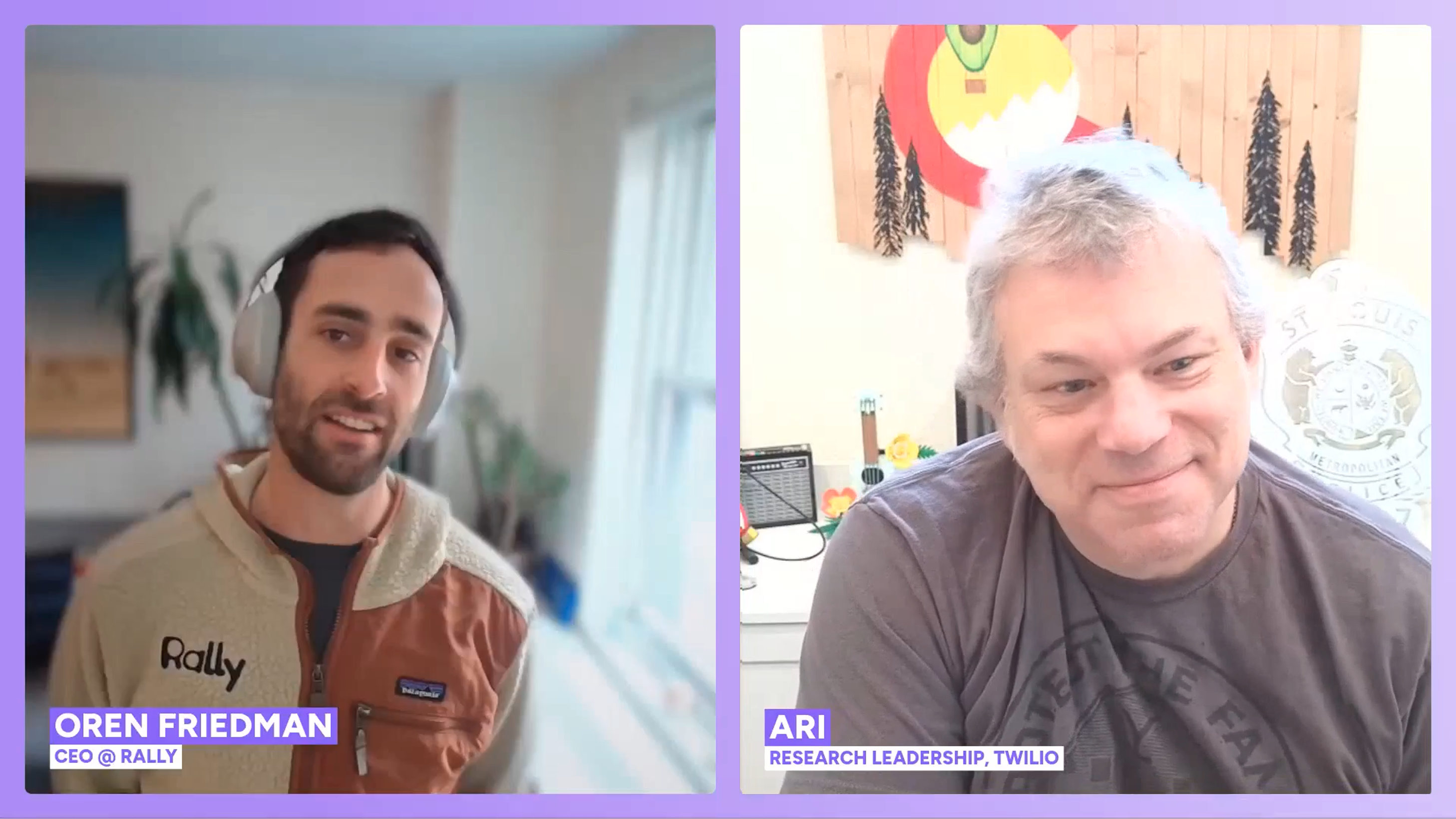
Who is Ari?
I got my start probably very different than almost everybody else. I was a police officer and detective for 10 years before getting into research. A criminal investigator turned customer investigator.
After I finished my doctoral program, I went into organizational psychology and corporate learning programs and I just didn’t like it.
Through a serendipitous turn of events, I ended up doing work in consumer packaged goods and market research, which led to building a research practice for Twitter’s data business. I was researcher number one for that, before it became what it is today.
Then I built research and insights teams for Panasonic and GTM Hub. I also did a stint at Indeed. Today, I’m at Twilio, where I lead Research and Research Operations for a big section of the business.
It’s really exciting. I’m seeing a lot of change – some of it terrifying, but I don’t think it’s a “sky is falling” situation.
From your perspective, where is AI currently being applied in research?
I think we’re seeing AI being used in a lot of different areas. People are experimenting across the research process: on the front end (like writing research questions), during research itself (customer- or participant-facing tools), and on the analysis side.
At Twilio, we use AI on the repository side to make it searchable, to ask questions, and pull valuable insights. I see a lot of value there. I’ve also experimented with AI tools that conduct interviews. I see some value, but those tools won’t replace a good human interviewer. They can’t dig deep, pick up on nuance, or understand context the way a human can.
One major issue is fraud, especially with panel participants that are unvetted. I’ve seen higher rates of fraud and poor-quality responses when using AI interview tools. AI can analyze huge datasets much faster than a human, but it’s not perfect. It misses things. We’ve all heard about hallucinations. They’re real.
If I were going to double down on one use case today, it would be on the administrative and back-end side of research not on participant-facing tasks. But that could change.
A year or two ago, did you expect AI to get this far? Where are we in the arc?
If you go back two years, this wasn’t even a glimmer on most people’s radar. Some folks were working on language models or NLP tools, but most of us were coding our interviews and using basic tools.
It’s important to remember how quickly the future can shift. Think about COVID. No one could have predicted the impact.Research is going through a major reckoning and not just because of AI.
Twenty years ago, software came in a box. The risk of being wrong was very high. Today, you can roll back a feature in seconds. But research didn’t evolve with that pace.
The research team of the future will be three things: faster, cheaper, and good enough.
We tend to over-index on rigor. That’s why AI tools are rejected by some in the academic world. They’re not “rigorous” enough. And sure, I’m not going to use an AI tool for pharmaceutical research today. But for usability testing? Sure.
What is the role of the researcher in the future? How does democratization fit in?
The uncomfortable truth is that businesses pay for what they value and might not value having researchers doing research. That’s a democratization issue. I think we’ll see a future with fewer researchers and a need to empower others to gather evidence, generate insights, and connect the dots.
Researchers are moving from data collectors to data connectors. How we collect evidence is less interesting than how we connect it to what the business cares about. We’ve historically done a poor job of that. We produce decks, readouts, and they sit in a repository – basically a graveyard.
The researcher of the future will connect people with evidence and information they need to make better decisions, faster. AI helps with that. It can surface what’s already known inside the business from different data sources and fill in the gaps. Then, and only then, do we move to primary research.
We can’t keep doing things the old way where someone brings a question and we jump into a research project without seeing what we already know. That’s not the future.
How are you bringing this to life in your current role at Twilio?
We’ve made a clear distinction between two types of research: building the right thing and building the thing right.
Building the right thing is messy and requires deep context. That’s where researchers are essential.
Building the thing right (e.g., usability testing, iterative improvements) is something we’re enabling our design and product partners to do themselves. It’s based on risk.
We’ve also changed how we report insights. 98% of our work never makes it into a deck. We use topline, memo-based reports that share insights as we learn them. It’s a shift to abductive reasoning where we’re sharing evidence with the right level of certainty and starting conversations earlier.
We’re reframing research as strategic learning and helping businesses learn continuously.
It’s also important to understand incentives. If research slows down shipping, product teams will ignore it. They’re incentivized to ship.
How can researchers lead in an era of AI and automation?
The first step is to reposition yourself. Most researchers generate insights. Fewer are seen as advisors. You want to be a consigliere – like in The Godfather – or the right hand of the king/queen in Game of Thrones. Trusted advisors.
Too often, researchers are treated like kiosks at McDonald’s: “I’ll take six interviews, a survey, and three usability tests.” Early on, researchers try to show value by producing outputs, not outcomes. That’s a problem.
To drive value, researchers need to act like credible experts and be the “doctors” of the business. We diagnose and prescribe. We don’t take orders. We should debate what we’re trying to learn, not what methods we’ll use.
We also need to stop acting as a surrogate function, just doing research because designers or PMs don’t have time. If we want to be seen as strategic, we have to act strategic. Stop asking for a seat at the table – just pull up a chair. Don’t ask for permission. Ask for feedback.
How can researchers deliver insights with perspective while remaining unbiased?
Researchers are often brought in to provide an unbiased perspective. But does true objectivity even exist? Everyone has bias. The key is to provide insights with a point of view, but remain open to changing it when new evidence appears.
That’s what researchers are trained to do. And that’s the core of what makes them essential: they can hold strong opinions, but adjust them when the facts shift.
What does it look like to operate with a point of view?
If two VPs come to you, one says, “Hey, I found this problem. What should I do?” and the other says, “I found this problem, here’s where we’re trying to go, here’s my proposed solution, here’s the evidence, and here are the risks” – which one do you trust more?
It’s the one with a point of view.
Researchers often present data and say, “Do what you’re going to do.” But if you want to make an impact, develop a perspective, back it with evidence, and be willing to change it.
Two years ago, I was anti-democratization. Today, I’m very pro. Why? Because new evidence showed that’s the path forward.
When it comes to democratization, people often think it means everyone should do all research all the time. I don’t believe that. But I do believe every PM, designer, and researcher should know how to do five basic methods: interviews, desk research, usability tests, observation, and basic surveys.
Those five, done well, are enough for a long, successful research career.
Also, people misuse the word “strategic” a lot. All research is strategic because strategy is just an intense focus on what matters most. A usability test with five people can be just as strategic as a pricing study with a broader business impact.
What changed your mind about democratization? How should we be layering AI into that thinking?
We’re being asked to do more with less. Teams are shrinking and we won’t bounce back to the size we were. So research has to be faster, cheaper, good enough.
AI is part of that shift. We should always be looking for ways to reduce cost and increase impact. That includes applying AI to repositories and, in the future, maybe to interviews like win/loss.
My shift toward democratization came from realizing research isn’t about data collection. It’s about how we use knowledge to create impact within the business. You could design the perfect, rigorous study. But if it doesn’t match product timelines, they’ll ship without you. And you’ll be too late. So build your process around how the business operates. Understand what the business needs. Respond, don’t react.
When new researchers join, they want to show value. They jump into a project. But the better approach is to pause, learn how the organization operates, and design research that fits into those workflows. That’s how you stay relevant and impactful.
How do researchers reposition themselves to be more impactful?
We’ve been having the wrong arguments for a long time. You run a study and the insights match what product wants, everyone’s happy. If the insights contradict product’s plan, suddenly the research is wrong. You’ll hear things like, “You only did six interviews,” or “Qual is weaker than quant.” The researcher of the future avoids these arguments entirely.
There are three types of arguments:
- Blame: “Why did you only do six interviews?”
- Value: “Qual is weaker than quant.”
- Decisions: “What are we going to do with this learning?”
The last one is the only one worth having.
Sometimes you need more evidence. Sometimes less. It depends on the risk. But arguing about how the sausage is made is a waste of time. Most stakeholders don’t fully understand qualitative or quantitative research. And that’s fine. Our job is not to argue methods, it’s to help make decisions.
Are UX researchers becoming redundant?
In their current state, yes. Companies want more people doing research not just dedicated researchers.
Where researchers have a leg up is in enablement. Helping others do quality research. That includes training, tooling, support, and evaluating new tools – especially AI tools. We’re well suited to guide that.
If a company procures an AI tool without a researcher involved, they risk flooding their repo with garbage. And bad data is worse than no data.
Additionally, researchers are becoming redundant in how we’ve always worked. But there’s a huge opportunity to reinvent how we work. Think of it like typing pools in the 1950s. Those jobs disappeared, but people didn’t. Their roles evolved. That’s what’s happening to research. It’ll happen to design too.
What are some non-obvious superpowers you’ve brought from your detective background?
One big one is abductive reasoning. Rather than following a linear path (start with a question, end with a deliverable), it’s about continuous learning. You gather evidence, build the most likely explanation, present your best case, and move on.
Another is how you dig for answers. I call it the “backhoe vs. shovel” analogy. You could dig a massive hole with a backhoe trying to find a gold coin in the yard but that’s inefficient. Shovel strokes let you learn and pivot. They bring stakeholders into the learning process. Stakeholders are your metal detector. They help you get closer to the answer without digging a giant hole.
Any quick hacks for using AI in research?
A few:
- Don’t test AI tools with company data without approval. Always run them through a security review.
- Test them outside of work using dummy projects, then assess them critically especially for recruiting and data quality.
- Measure twice, cut once. A killer prompt makes all the difference. You can reuse well-written prompts for discussion guides or summaries.
- Only use AI tools if they save time. If you have to double-check everything, you’re not gaining much. The output has to be useful and accurate.
- Test tools in real-world conditions. Don’t base a business case on cost alone. If the data is bad, you’re just polluting your repo.
How do you talk to non-researchers about the risks of synthetic user data?
I’m not a fan of synthetic data for research. Maybe for generating hypotheses but not for decision-making.
There are a few big problems:
- The problem of induction. Using the past to predict the future doesn’t always work. Like the Thanksgiving turkey who assumes the farmer is his friend until the day before the holiday.
- Humans are emotional. We make emotional decisions and justify them with logic. Synthetic users can’t replicate that.
- Lack of transparency. Providers won’t show you how they create the synthetic data. You’re trusting a black box.
Could it be right sometimes? Sure. But so is a broken clock twice a day.
When talking to stakeholders, tie your argument to what the business cares about:
- Growth
- Value
- Adaptability
- Risk
- Speed
Synthetic data increases risk. That’s enough to get their attention.
What lifts has AI removed from research so far, and where is it going next?
The biggest benefit from AI right now is speed. It’s not perfect. It doesn’t capture every quote or pull every insight. But it’s getting better fast. In the six months we’ve been using AI in our repository, it has improved exponentially.
Product and engineering don’t want to wait for decks or reports. They want to move. So the faster we can get to useful insights and a point of view, the better.
I’m also excited about having a perplexity-like interface where anyone in the company can ask a question, get an answer, and see supporting evidence. That’s a game-changer for research velocity.
There’s also value in AI as a thought partner or assistant especially for administrative tasks, but the long-term value is in helping everyone access insights, quickly.
What hard skills will researchers need to become better data connectors?
First, both quant and qual are valuable. You can’t understand the “how do we stop the bleeding” part of churn from data alone, you need the why. And qualitative research gets you that.
The five methods I always come back to are:
- Interviews
- Desk research
- Usability tests
- Observation
- Basic surveys
Master those and you’re in a strong place.
That said, it’s also helpful to understand things like statistical significance, sampling, margin of error – even if just enough to push back or explain why they may not be relevant. But more important than learning another method is learning how to influence decisions. How do you position your findings in terms the business cares about?
And here’s the hard truth: nobody wants “research.” What they want is the outcome of research – the confidence to make a decision that drives growth, value, adaptability, reduced risk, or speed.
Any final thoughts or parting advice?
We’re here to cross the swamp, not fight every alligator. Choose your battles. You don’t need to solve everything today.
With AI, layoffs, shifting orgs, it can feel overwhelming. So focus on what matters most. Take an abductive approach to evolving research inside your org. Look at the evidence, make your best case, and iterate.
Change won’t happen overnight. But part of the game is adapting as it does.
Connect with Ari
If you enjoyed Ari’s AMA:
- Follow him on LinkedIn and say hello!
- Get the best blueprint for strategic research.
- Take Ari’s research course.
Thank you, Ari!
We’ve been following Ari and his work for a long time and were so excited to have him join our AMA series. We greatly appreciate his willingness to share his time, energy, and expertise with us. If you’d like to watch the full AMA, follow this link.